Freedom to Experiment refers to allowing every actor in a system as much freedom as possible to choose the path—or hypothesis—that, on the basis of their experience and perspective, is most likely to achieve their desired outcome.
You can think of it as a sports team on the field. Each member of the team needs to sense what is happening around him or her and respond quickly and strategically, in order to contribute to winning the point.
With that freedom to experiment comes the expectation that these actors will treat their decisions and actions as an experiment (versus assuming their decision was “right” and any failure was due to some external factor) and to honestly assess and learn from their success or failure. After a game — win or lose — a championship team will review the game tape to reflect on their individual and collective actions and how they contributed to the ultimate result. This freedom-plus-accountability accelerates the rate at which a team, community, or whole ecosystem can learn.
Why is it important to maximize freedom to experiment?
Freedom to experiment transforms how diverse participants are encouraged and enabled to show up in learning discussions. An experimentation posture invites curiosity of the group and individuals participating in it. It invites stories and data that may challenge dominant hypotheses, allowing for adjustments or the emergence of new hypotheses. Robust and repeated experimentation provides us with a larger dataset on which we can base our findings, increasing our confidence in these findings.
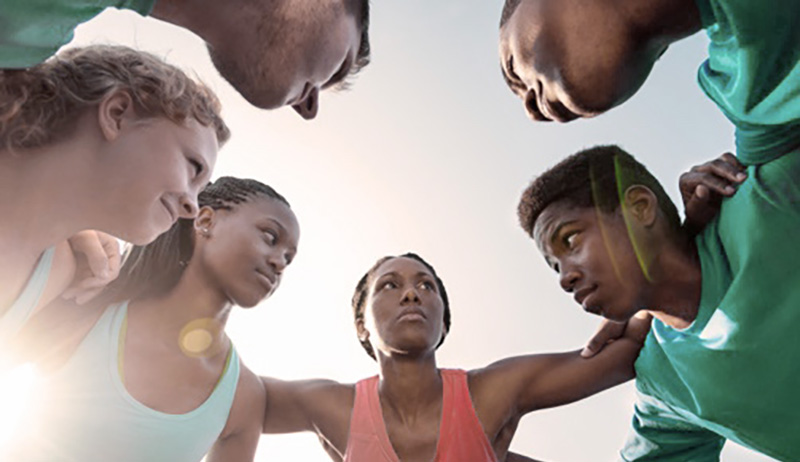
What does this principle look like in practice?
The presence or lack of an environment that encourages experimentation can manifest itself in a number of ways. Here is what we have observed:
- If it’s present: There is a clear distinction between the goal (the “what”) and the strategy or plan to get there (the “how”) and people in the whole system know that they are able, if not encouraged, to experiment with different approaches and/or to shift gears if something is not working. There is an expectation that they and everyone else will learn from these experiments, and the system takes the time to notice and reflect on results.
- If it’s missing: Members of the system are expected to align their resources and/or follow a prescribed set of expectations about how to implement a strategy or initiative. There may be a lag time between early indicators that something is not working and when people are willing to speak up or hear bad news from others, so that the approach can be adjusted or even reconsidered. This lag time may be proportional to the investment made in developing a strategy or solution. The more “right” that path is perceived, the harder it can be to challenge it.
How Emergent Learning practices support this principle
The practices of Emergent Learning work together to support experimentation:
- Framing Questions invite people into a shared experimental mindset. They communicate that there is not one right answer and that it is our collective job to learn and discover together.
- “If/Then” Hypotheses help us make the cause/effect thinking behind our decisions explicit. They remind us that the strength of our thinking is measured by the results we achieve.
- Before and After Action Reviews bookend action to turn it into a learning experiment. They invite everyone involved in the action to participate in the experiment.
- Emergent Learning Tables help groups collect and reflect on the results of all of the ‘experiments’ they have done so far. They allow each actor to come away with an idea that’s relevant to them and test it out (rather than holding a goal of aligning around a single idea).
- Learning Logs help us capture results and insights from experiments while they are fresh. They help us keep everyone on the team informed about our experiments, results, and insights.
How can we support experimentation in everyday conversation?
Emergent learning questions, in themselves, support experimentation. In everyday conversation, Emergent Learning practitioners learn to ask questions in real-time that reinforce a culture of experimentation. Questions like:
- What have we learned from similar situations in the past?
- What are our intended results?
- What is our hypothesis (if/then)?
- What were our actual results vs. intended results?
- What caused our results?
- Are there any counter-examples (i.e., where we have seen different results)?
- If we could turn the clock back, what would we do the same or differently?